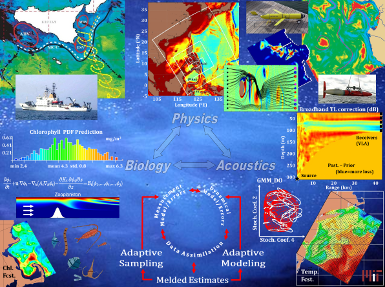
Physical and Interdisciplinary Regional Ocean Dynamics and Modeling Systems
Our research includes: quantifying of ocean features and dynamics, multi-scale numerical modeling, and uncertainty quantification and assimilation schemes. Recent results include high-order hybrid Finite-Element schemes for physical-biological dynamics at shelfbreaks (bottom left), rigorous nonlinear and non-Gaussian data assimilation using our GMM-DO filter and smoother (bottom right) and exact path planning for swarms of underwater vehicles using level-set equations (top right).
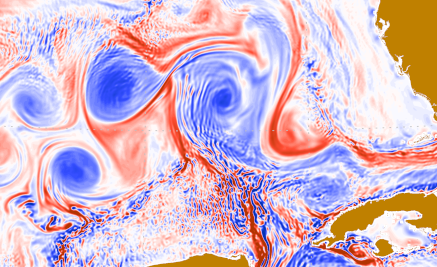
Advancing Gulf of Mexico Operational Forecasting with Application to Fisheries, Industry Safety, and Natural Hazards (GOFFISH)
This project aims to achieve measurable and significant improvements in short- to medium-range (1- to 10-day), subseasonal, and long-range (3- to 6-month) prediction skill of sustained continuous operational forecasts of Gulf of Mexico (GoM) ocean dynamics.
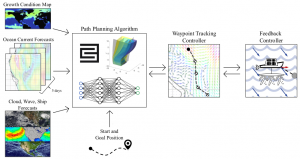
Affordable Gigaton-Scale Carbon Sequestration
In this research we combine of probabilistic ocean modeling and control approaches such as reachability with machine learning for optimal path-planning algorithms in strong uncertain dynamic fields. Research tasks include: (1) Realistic Data-driven Ocean and Platform Modeling and Simulation; (2) Optimal Path Planning with High-Dimensional Reachability, Plan Adaptation and Learning; (3) Learning a Final Value Function for long-term Anticipation and Deep Reinforcement Learning Algorithms.
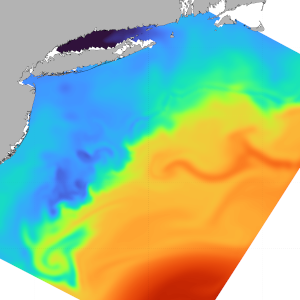
Compression and Assimilation for Resource-Limited Operations
The primary goal of this project is to enable effective use of ocean forecast data on forward operating platforms with limited communications and computing resources. The aim is to mature and assess promising approaches to this problem for subsequent investment leading to transition of the developed capabilities to operations. Our MIT-MSEAS research will consist of four research thrusts involving reduced order modeling (ROM): i) decomposition of deterministic and probabilistic forecasts for efficient compression, reduction and reconstruction; ii) machine learning for reduced-order modeling and forecasting; iii) adaptive and data-assimilative reduced-order modeling and forecasting; and iv) multi-resolution and multi-dynamics ROMs.
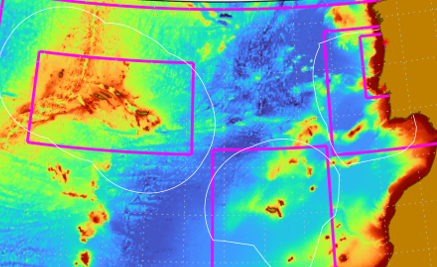
Knowledge and Data from the Deep to Space
(K2D)
The deep sea is currently at the heart of the last remaining, big knowledge frontier. Information about the deep sea is critical to close the loop from oceans to near space. The breath of scales is large, but the challenges are mostly technological. Inhospitable and extreme environments are the obstacle, where the rules are radically different. Efficient exploration, monitoring and data communication systems for deep sea are essential to exploration.
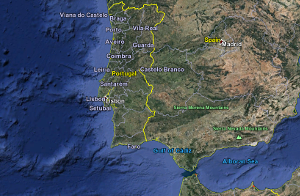
Intelligent Observing and Multiscale Modeling for Ocean Exploration and Sustainable Utilization
(Portugal)
For intelligent ocean exploration and sustainable ocean utilization, the need for smart autonomous underwater vehicles, surface craft, and small aircrafts is rapidly increasing. Applications include scientific studies, solar-wind-wave energy harvesting, transport and distribution of goods, naval operations, security, acoustic surveillance, communication, search and rescue, marine pollution, ocean cleanup, conservation, fisheries, aquaculture, mining, and monitoring and forecasting. Designing optimal paths leads to cost savings, longer operational time, and environmental protection.
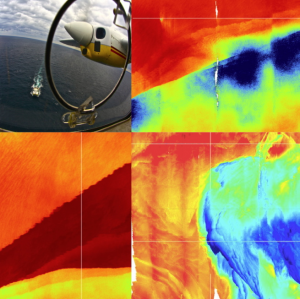
Machine Learning for Submesoscale Characterization, Ocean Prediction, and Exploration (ML-SCOPE)
Our long-term goal is to obtain machine-intelligent modeling systems that seamlessly integrate stochastic ocean dynamical models and their multi-fidelity representations with Bayesian and generative learning from data-model misfits, to construct improved ocean models with more accurate parameterizations and discover invariances or differential equations, over a range of spatial and temporal scales. We will use and vastly extend stochastic dynamic reduced-order methods, Bayesian GPs, adaptive DL schemes, and generative networks. Ultimately, our symbolic interpretation of ML models into emergent dynamical and constitutive relations would further compress knowledge relative to deep networks, thus extending outside the range of the training data.
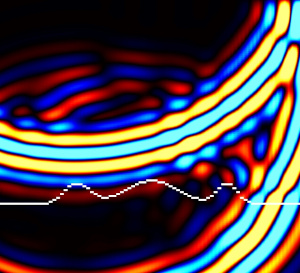
Dynamic Environmental Estimation, Prediction, and Acoustic Inference (DEEP-AI)
The main goal for our project is to further develop, implement, apply, and validate theory, algorithms, and computational schemes for dynamic environmental estimation, prediction, and acoustic inference (DEEP-AI). The specific research thrusts are to: (i) Predict and characterize underwater sound propagation PDFs, (ii) Assimilate the sparse acoustic and oceanographic data, (iii) Learn and discover acoustic parameterizations, model improvements, new processes, and most informative observation needs, and (iv) Develop efficient computational methods for the above prediction, assimilation, and learning.
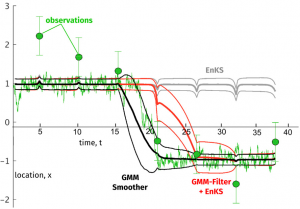
Interdisciplinary Nonlinear Bayesian Data Assimilation
Our long-term goal is to: generalize, develop, and implement our stochastic dynamically-orthogonal decompositions and nonlinear Bayesian filtering and smoothing schemes for principled probabilistic predictions and predictability studies of physical-acoustical-biogeochemical-sea-ice dynamics, and for interdisciplinary nonlinear Bayesian data assimilation, adaptive sampling, and quantification of observation needs for naval operations.
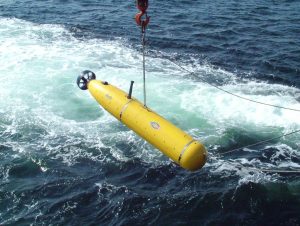
Local Stochastic Prediction for UUV/USV Environmental Awareness for Applied Ocean Sciences
We plan to collaborate with Applied Ocean Sciences (AOS) to help designing and delivering a compact system to assess local uncertainties and track the evolution of the maritime environment around unmanned platforms at sea. Such a system can run both at control centers and on-board Underwater and Surface Unmanned Vehicles (UUV/SUV) under different network bandwidth constraints. The system uses the Navy ocean forecasts for initial environmental guesses and outlooks up to 2 weeks (or more in future generations) and then implements a Reduced Order Model (ROM) derived from Dynamically Orthogonal (DO) solutions to deliver a local uncertainty picture (for the next 24-48 hours).
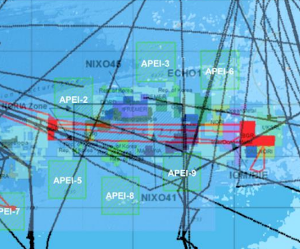
The Potential Effects of Deep Sea Mining on Deep Midwater Communities in the CCZ
Understanding the scale of deep-sea mining’s effects is paramount to understand their ecosystem consequences. There is no doubt that deep-sea mining will kill organisms and permanently alter habitats. Seafloor disturbance studies clearly show that benthic communities don’t fully recover even after several decades. Further, much of the fauna relies on the nodules themselves which take millions of years to form. These effects over hundreds of meters to a few kilometers may not comprise a substantial ecosystem impact given the scale of abyssal habitats, but effects over hundreds to thousands of kilometers would surely be defined as serious environmental harm. There is a clear need to use sediment plume models to estimate the scale of mining impacts in the midwater realm.
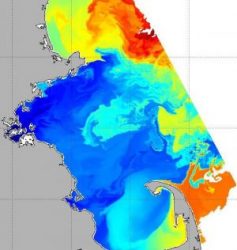
Plastic Pollution in the Oceans: Characterization and Modeling
Since the 19th and early-20th century, plastics have become ubiquitous in the world. Plastics have outgrown most man-made materials: their global volume production has surpassed that of steel production in the late 1980s (Fernandez et al., 2018). The MIT Environmental Solutions Initiative (MIT-ESI) aims to tackle this challenge through the expertise of our interdisciplinary faculty, ranging from materials, to manufacturing and design, to smart sensing and advanced computational modeling and data-driven learning. Through our approaches and collaborations, our long-term goal is to develop a plastic free environment that will ensure the health of our planet.
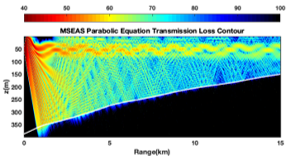
Wide Area Ocean Floor Mapping
Our primary goal is to further develop, implement, apply, and validate theory and schemes for a prototype Wide Area Ocean Floor Mapping system. Our specific objectives are to: (i) Characterize and predict underwater sound propagation uncertainty/distributions due to inexact bathymetry fields and ocean environmental and seabed uncertainties, and (ii) assimilate limited acoustic and oceanographic data for the joint principled Bayesian inversion of environmental and acoustical fields, and for the corresponding rigorous estimation of bathymetry.
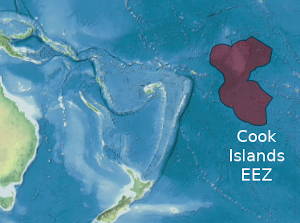
Deep Sea Mining: Modeling, Observing and Quantifying Risks
Our research aims to address the outstanding questions surrounding deep-sea mining sediment plumes as this underpins all understanding and prediction of the biological response to deep-sea mining activities, and furthermore provides the foundation for the design of marine protected areas and other regulations. Limited resources have been available to understand this issue, which policymakers have identified as being of urgent need. Over the past fifteen years, only two sets of model results have been obtained (one from a European researcher and another from a European contractor) and they differ significantly in their predictions.
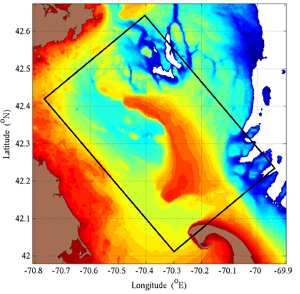
Bayesian Intelligent Ocean Modeling and Acidification Prediction Systems (BIOMAPS)
The overarching goal of this project is to develop and demonstrate principled Bayesian intelligent ocean modeling and acidification prediction systems that discriminate among and infer new ocean acidification (OA) models, rigorously learning from data-model misfits and accounting for uncertainties, so as to better monitor, predict, and characterize OA over time-scales of days to months in the Massachusetts Bay and Stellwagen Bank region.
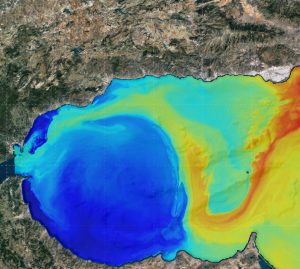
Coherent Lagrangian Pathways from the Surface Ocean to Interior (CALYPSO)
Describing and quantifying the truly three-dimensional and time-dependent transports of ocean properties from the surface ocean to the interior is a fascinating observational, theoretical, and modeling challenge. The CALYPSO initiative addresses this challenge, with a focus on the southwest Mediterranean Sea region. Our goal is to develop novel efficient four-dimensional Lagrangian analysis theory and methods, and apply and expand our capabilities in multi-resolution multi-disciplinary ocean modeling, uncertainty, predictability, and Lagrangian–Eulerian data assimilation, to predict and characterize multiscale ocean transports, coherent structures, and subduction/stirring/mixing processes, and optimally guide ocean platforms towards the most informative observations.
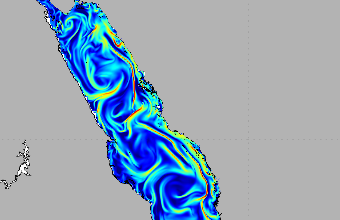
Red Sea Initiative
Our research focus will be the Lagrangian connectivity of marine ecosystems and sea hazards due to natural or made-made Lagrangian material transports. Specifically, we plan to further develop and apply our new theory and schemes for (i) the study and quantification of biogeochemical coherent structures and Lagrangian connectivity of marine ecosystems, and (ii) the study and mitigation of sea hazards due to stochastic advection and Lagrangian material transports including marine contaminations and spills.